Looking into the future: Predictive analytics in supply chain management
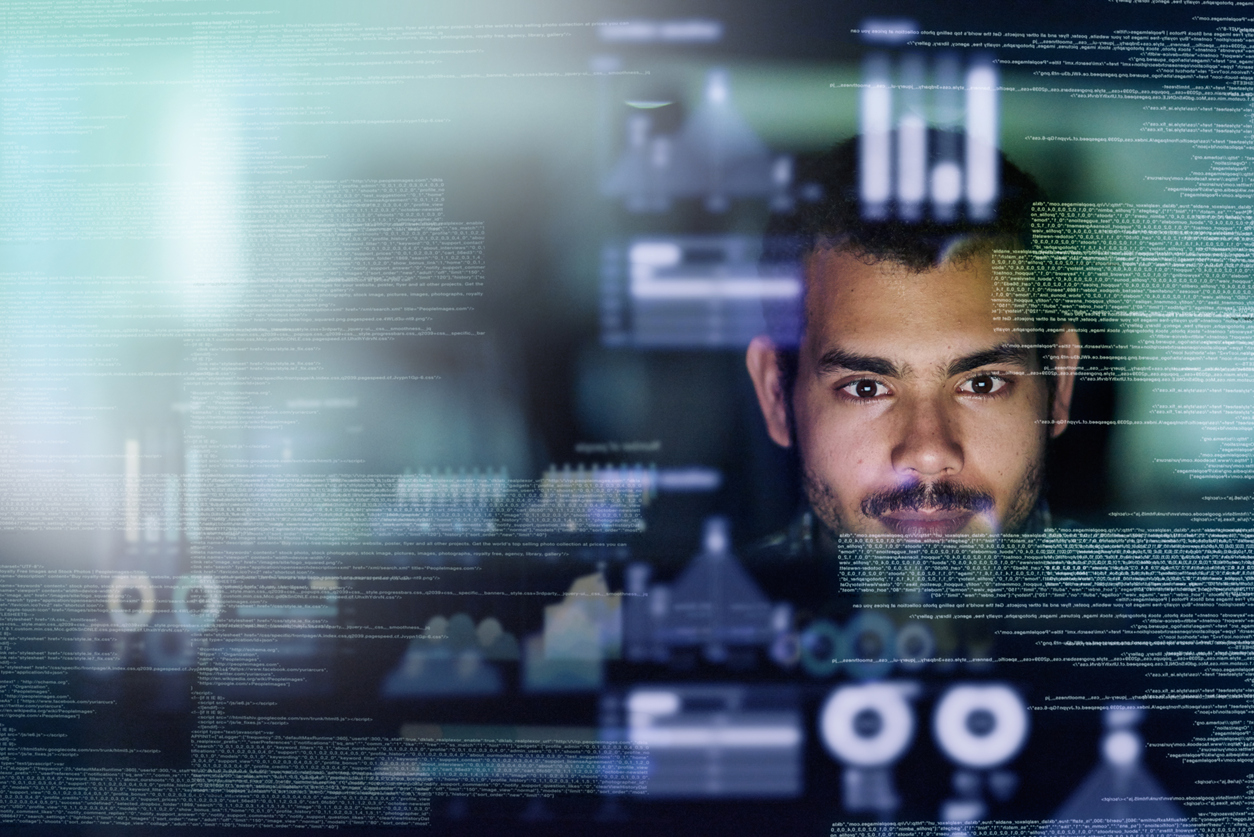
As a branch of business analytics, predictive analytics is focused on making projections about relevant events in the future. Although not known by this particular term, the discipline itself has long been used to manage supply chains.
The VMI process is a good example of a long-standing predictive analytics approach. When regulating supply using VMI, the customer defines inventory thresholds that suppliers are not allowed to exceed or fall short of over the course of their deliveries. The customer first informs his supplier about the current inventory level and the expected gross quantities required over a defined time period. After planning the production and transport of the goods in question, the supplier then informs the customer about the planned deliveries. If he has a VMI monitor, the customer can use it to compare his demand forecast (taking into account the current stock level) with the supplier’s planned deliveries, including goods already in transit.
The result is the anticipated future inventory level over the course of the relevant period, based on the data available. Should the defined inventory thresholds be jeopardized at any given time, the monitor dispatches a warning message to all the parties involved so that they can take action to remedy the situation (see diagram).
Predictive analytics: identifying and solving problems at an early stage
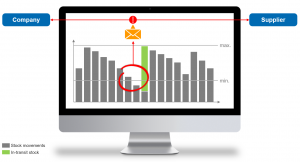
The software calculates future inventory developments on the basis of the customer’s expected demand and the capacity situation of the supplier. If necessary, it alerts both parties to critical situations that lie ahead. Ideally, these critical situations are so far in the future that the problem can be solved using normal planning procedures, thus avoiding expensive special measures, delays or even production downtimes.
An example: If the problem is caused by insufficient production capacity on the part of the supplier over a certain period, due to the high number of orders, the customer can consider requesting a partial delivery of his demand at an earlier date than originally planned. Accepting this temporary infringement of the maximum inventory threshold may alleviate the problem or prevent it entirely.
This example demonstrates the basis of predictive analytics: identifying critical problems early enough for countermeasures to be taken to safeguard the company from increased costs or other damage.
The challenge: using predictive analytics in globally networked supply chains
The application of predictive analytics is particularly difficult in supply chains networks that are becoming increasingly interconnected and global by the day. This is because the coordination of demand and capacities between customers and suppliers is a hugely complex task for all concerned. In addition, the process involves so many players and key variables, that an overall optimization is infeasible.
All companies involved have their own separate planning and data storage systems, and the individual business objectives of these players are often incompatible with end-to-end processes and full transparency of data. Realistically, the multi-tier scenarios and systems that are frequently talked about in expert circles and specialist media are still a vision of the future.
In view of all this, the only viable way of consistently employing predictive analytics in supply chain networks as described above, seems to be to set up platforms or business networks between the individual stages/tiers of the chain. These then collect and analyze the supply chain data centrally for a large number of companies (see diagram below). Airbus has already opted for this method with AirSupply.
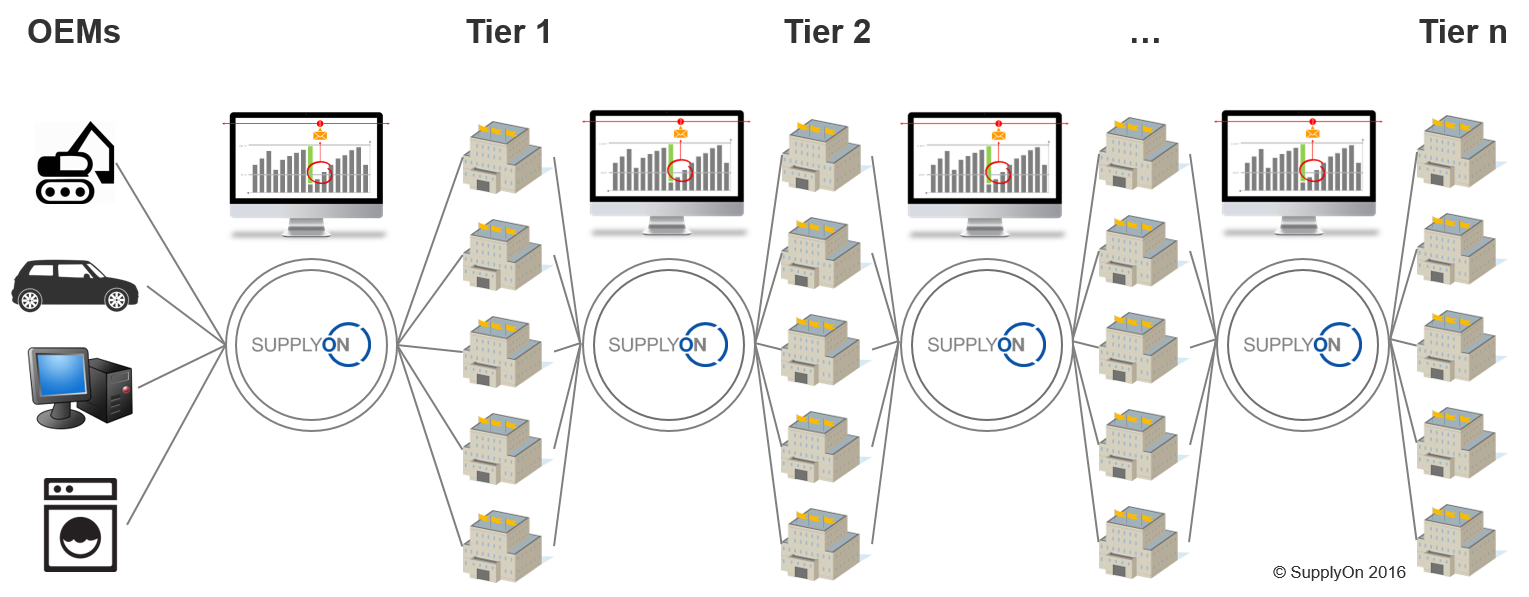
A vital prerequisite for the viability of this approach is the end-to-end digitization of the data flow and direct processing of all the data necessary for a comparison of target and actual values by the system without any manual effort, facilitating – when combined with configurable alerting mechanisms – an uninterrupted flow of goods along the entire supply chain.
Those companies that take on the digitalization of this process early on and secure a head start will almost certainly be able to turn this into a real competitive advantage.